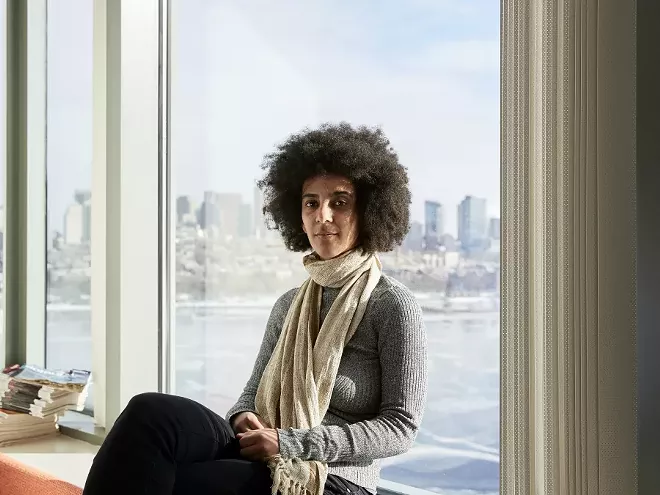
By STEVE LOHR
© 2017 New York Times News Service
What vehicle is most strongly associated with Republican voting districts? Extended-cab pickup trucks. For Democratic districts? Sedans.
But what is surprising is how researchers working on an ambitious project based at Stanford University reached those conclusions: by analyzing 50 million images and location data from Google Street View, the street-scene feature of the online giant’s mapping service.
For the first time, helped by recent advances in artificial intelligence, researchers are able to analyze large quantities of images, pulling out data that can be sorted and mined to predict things like income, political leanings and buying habits. In the Stanford study, computers collected details about cars in the millions of images it processed.
“All of a sudden we can do the same kind of analysis on images that we have been able to do on text,” said Erez Lieberman Aiden, a computer scientist who provided advice on one aspect of the Stanford project.
For computers, as for humans, reading and observation are two distinct ways to understand the world, Lieberman Aiden said. In that sense, he said, “computers don’t have one hand tied behind their backs anymore.”
Text has been easier for AI to handle, because words have discrete characters — 26 letters, in the case of English. That makes it much closer to the natural language of computers than the freehand chaos of imagery. But image recognition technology has improved greatly.
By pulling the vehicles’ makes, models and years from the images, and then linking that information with other data sources, the Stanford project was able to predict factors like pollution and voting patterns at the neighborhood level.
In the end, the car-image project involved 50 million images of street scenes gathered from Google Street View. In them, 22 million cars were identified, and then classified into more than 2,600 categories like their make and model, located in more than 3,000 ZIP codes and 39,000 voting districts.
But first, a database curated by humans had to train the AI software to understand the images.